
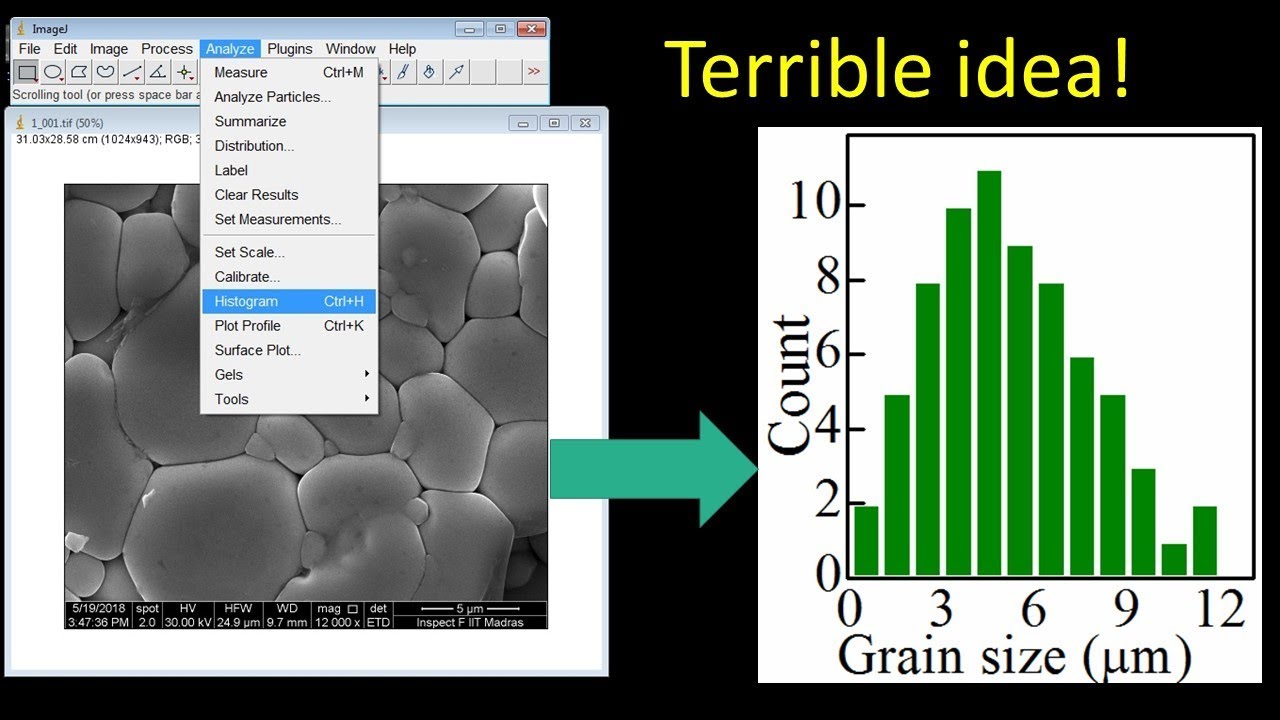
Scientists have thought for decades that MS is primarily characterized by chronic inflammation and loss of the myelin sheaths surrounding the axons in the CNS ( Stadelmann et al., 2008). Another important example is multiple sclerosis (MS). This has important consequences for developing novel treatment strategies against age-related impairments in memory ( Abbott, 2012). Since then, the concept has emerged that age-related impairments in memory are not linked to hippocampal cell death but to specific and relatively subtle synaptic alterations in the hippocampus and prefrontal cortex ( Morrison and Hof, 1997 Hof and Morrison, 2004 Morrison and Baxter, 2012). However, stereologic cell counting has contradicted much of this work ( Gallagher et al., 1996 Rapp and Gallagher, 1996 Wickelgren, 1996). For example, based on several studies, until 1996 it was thought that the “benign forgetfulness” of people over 65 is due to the death of neurons in the hippocampus, a structure within the central nervous system (CNS) known to be critical to learning and memory ( Wickelgren, 1996). Stereologic cell counting has had a major impact on the field of neuroscience over the past 20 years.
#Count nuclei imagej manual#
Thus, at present, stereologic cell counting with manual decision for object inclusion according to unbiased stereologic counting rules remains the only adequate method for unbiased cell quantification in histologic tissue sections. The results demonstrate that the current automated methods suffer from lower detection rates and higher false-positive rates than are acceptable for obtaining valid estimates of cell numbers.

Of these methods, FARSIGHT performed best, with true-positive detection rates between 38 and 99% and false-positive rates from 3.6 to 82%. The evaluation compared the numbers and locations of cells identified unambiguously and counted exhaustively by an expert observer with those found by three automated 3D cell detection algorithms: nuclei segmentation from the FARSIGHT toolkit, nuclei segmentation by 3D multiple level set methods, and the 3D object counter plug-in for ImageJ. The image data used in this study were 3D microscopic images of thick brain tissue sections prepared with a variety of commonly used nuclear and cytoplasmic stains. We present an evaluation of the performance of modern automated cell detection and segmentation algorithms as a potential alternative to the manual approach in stereologic cell counting. Reliance on visual inspection forces stereologic cell counting to be very labor-intensive and time-consuming, and is the main reason why biased, non-stereologic two-dimensional (2D) “cell counting” approaches have remained in widespread use. A major bottleneck in stereologic cell counting is that the user must manually decide whether or not each cell is counted according to three-dimensional (3D) stereologic counting rules by visual inspection within hundreds of microscopic fields-of-view per investigated brain or brain region. Stereologic cell counting has had a major impact on the field of neuroscience.
